Supervised machine learning for ultracold atoms in speckle disorder
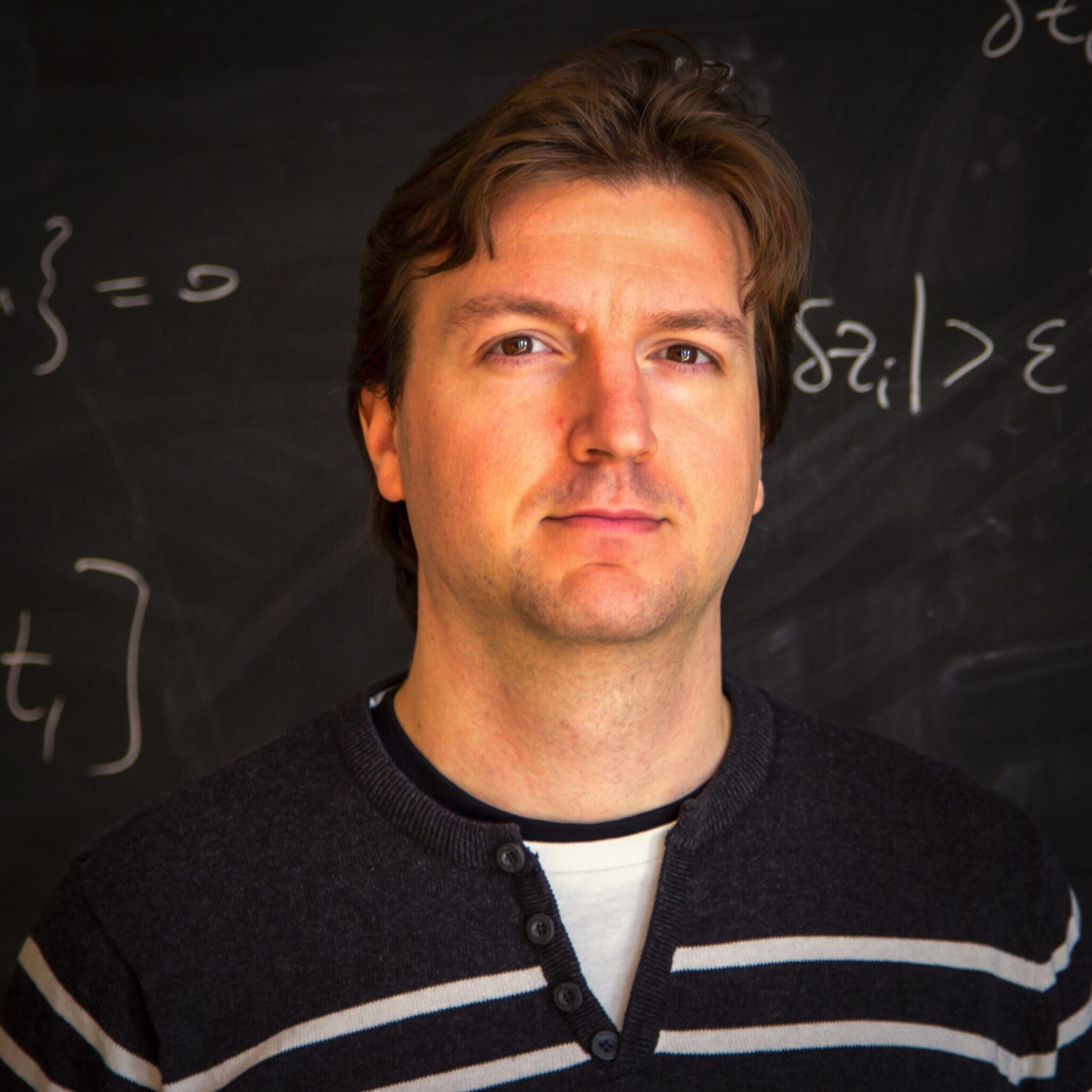
In this work, we consider the use of supervised machine techniques to address quantum many-body problems relevant for experiments performed on disordered ultracold atoms.
After a broad introduction on supervised machine techniques, I will show how a deep neural network can be trained to make accurate predictions on the low-lying energy levels of noninteracting atomic gases in optical speckle patterns. Interestingly, we find that the neural network is remarkably resilient to the noise added in the training (here, synthetic) data, suggesting that, in the long term, cold-atom quantum simulations could be used to training neural network to solve hard problems that cannot be efficiently addressed using classical computers as, e.g., the many-fermion problem.
I will also show recent results on the use of convolutional neural networks, usually employed in image recognition applications, to build a scalable neural network model that allows one to make predictions for system sizes larger than the ones employed during the training stage, providing us with a novel extrapolation technique. I will also highlight the use of transfer learning techniques to speed-up the learning process for large system sizes.