Intelligent Arxiv: Sort daily papers by learning users topic preferences
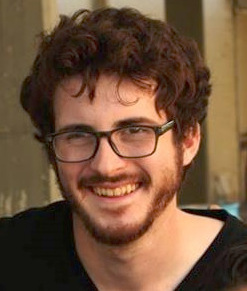
We model a scientific paper to be built as a combination of different scientific knowledge from diverse topics into a new problem. We apply then the (unsupervised) Machine Learning technique LDA to construct and extract topics from the corpus of papers. We obtain the topic weights of the available and new papers in the Arxiv, and determine each user preference in topics according to each user preference in papers.
This allows us to determine the personal preference on new papers according to their topics weight distribution. We have created the web interface IArxiv.org where users can read personally-sorted daily Arxiv releases (and more) while the algorithm learns his/her preferences. Yielding therefore a more accurate sorting every day. Current IArxiv.org version runs on categories astro-ph, gr-qc, hep-ph and hep-th.